Postdoctoral Fellows
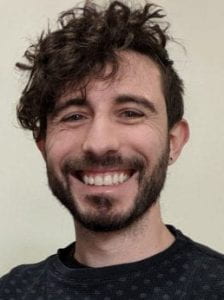
Vladislav Ayzenberg
Department of Psychology
Vlad is a developmental cognitive neuroscientist who is interested in understanding the mechanisms that support early developing perceptual abilities in human infants. Because measuring the underlying processes of the infant brain is incredibly challenging, Vlad uses biologically plausible computational models to explore what kinds of processes and developmental constraints may be sufficient to support infant perception. As a postdoctoral fellow with Dr. Michael Arcaro, he is starting to explore how early developing anatomical structures in the neonate visual system may scaffold perceptual abilities later in life.
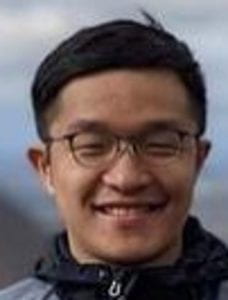
Chang-Yu Chang
Department of Biology
Chang-Yu Chang is a systems biologist who combines computational and experimental approaches to study the ecology and evolution of microbial communities. His past works focused on engineering the emergent properties of microbial consortia using directed evolution. With Dr. Corlett Wood, he is starting to work on host-associated microbial ecosystems and asks how the interplay between ecological interactions and genomic structures shapes the host phenotypes. Chang-Yu is originally from Taiwan.

Sunghye Cho
Linguistic Data Consortium
Sunghye Cho is a linguist who has extensive research experience in acoustic phonetics and fully automated analyses of natural speech data with large-scale speech corpora. She has examined various characteristics of natural speech produced by patients with language and speech impairments not only at the phonetic, prosodic levels but also at the lexical, semantic, and syntactic levels using cutting-edge technologies in natural language processing and automated speech detection systems. Her recent projects focus on identifying novel language and speech biomarkers of neurodegeneration and autism spectrum disorder and building automatic classification systems using those biomarkers.

Sam Dillavou
Department of Physics and Astronomy
Sam Dillavou is a physicist interested in the overlap between physics and machine learning, and how the two fields can inform and support each other. His projects include building physical systems that can perform machine learning tasks (learn) without a processor, studying complex systems like granular flows that have resisted understanding using standard statistical methods, and using machine learning to make experimental science easier and more accessible.

Andrew Koepp
Department of Psychology
Andrew is an applied developmental psychologist studying how young children learn to manage their attention and behavior in the classroom. He uses wearable accelerometers to capture children’s inattentive and impulsive behaviors, leveraging the objective and continuous recording of movement to understand daily fluctuations in children’s behavior. With support from Penn’s Data Driven Discovery Initiative, Andrew will apply supervised machine learning methods to evaluate which features of children’s movement (i.e., amount, intensity, onset, duration) best predict teacher ratings of children’s impulsivity and inattention. Andrew also looks forward to using unsupervised clustering techniques to identify temporal patterns of children’s behavior and network analysis to study social dynamics in the classroom.

Sarah Lee
Department of Linguistics
Sarah Hye-yeon Lee is a linguist who is interested in the interface between language and cognition. Her work takes an experimental, data-driven approach to understanding the relationship between language and non-linguistic conceptual structure, as well as to understanding the cognitive mechanisms that underlie real-time language processing. Her research uses a range of behavioral data (e.g. reaction time data, eye-tracking data) and corpus data. Sarah holds a Ph.D. in Linguistics from the University of Southern California.

Sergey Molodtsov
Department of Earth and Environmental Science
Sergey Molodtsov is a physical oceanographer who is interested in studying large scale ocean circulation and its role in the climate system. Such research always involves dealing with large observational and modelling oceanographic and climate datasets, e.g. remote sensing imagery, general circulation models output and in-situ observational oceanographic datasets. He is applying machine learning to identify different relationships between various components of the ocean and climate system. Sergey holds a Ph.D. in physical oceanography from Texas A&M University.
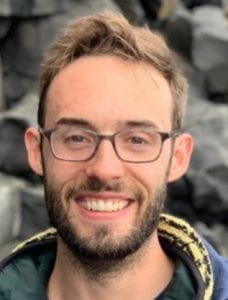
Kieran Murphy
Department of Bioengineering; Physics and Astronomy
Kieran is a physicist entranced by information theory, leveraging machine learning to track down information in general relationships through data. Recent projects have focused on developing methods to localize information: deconstructing complex systems into intelligible approximations — including the Mona Lisa and a simulated glass under shear — and switching around a standard representation learning method to isolate information out of mere groupings of data.

Erçağ Pinçe
Department of Physics and Astronomy
Erçağ Pinçe is an experimental biophysicist investigating the role of bacterial motility in establishing multispecies microbial communities. He addresses the questions of whether motile cells can alter swimming behavior in response to local hydrodynamical stresses and solve an optimal navigation problem within a complex and disordered environment. Can they adopt optimal navigation policies to become early colonizers and outcompete other microbial species? Erçağ tackles these questions by combining microbiology, high-throughput 3D cell tracking microscopy, and microswimmer modeling based on a reinforcement learning framework. Through statistical analysis of large tracking datasets, he characterizes the motility pattern of naturally isolated bacteria and sets interaction studies amongst different motile phenotypes to uncover multifaceted phenomena of bacterial navigation.

Carlos Schmidt-Padilla
Department of Political Science
Carlos is a political scientist that leverages applied data science methods and geospatial analysis to study the political economy of development in Latin America and in sub-Saharan Africa. Specifically, his research focuses on crime, health, human capital, migration, and policing. Carlos earned his doctorate in Political Science at the University of California, Berkeley, in August 2021.

Brynn Sherman
Department of Psychology
Brynn Sherman is a cognitive neuroscientist interested in human memory. In particular, she combines insights from human behavior, data-driven analysis of neuroimaging data, and computational models to understand how we use our memories to adaptively guide future behavior. In her current projects, she is taking empirical and computational approaches to understanding how sleep facilitates the integration of new memories with existing knowledge.

Adrien Thob
Department of Physics and Astronomy
Adrien is an astronomer interested in understanding the mechanisms that drove the formation of galaxies throughout the history of the Universe. Thanks to his multidisciplinary career with a background in telecommunication and data processing engineering, he routinely uses data science, high performance computing and software development in his research. Adrien holds a PhD in Computational Cosmology from the Astrophysics Research Institute of Liverpool John Moores University in the UK, and joined the University of Pennsylvania as a postdoctoral researcher after a first postdoctoral position at the University of Washington.
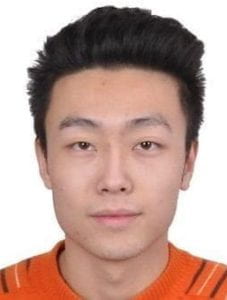
Jingye Yang
Department of Mathematics; Pathology and Laboratory Medicine
Jingye Yang obtained his Bachelor’s degree from Xi’an Jiaotong University, and later earned a Ph.D. in Mathematics from the University of Pennsylvania. He also held dual Master’s degrees in Applied Mathematics and Computational Science (AMCS) and Statistics from the University of Pennsylvania. As a postdoctoral researcher at the University of Pennsylvania and the Children’s Hospital of Philadelphia, Jingye Yang specializes in Mathematics and Machine Learning within the field of Bioinformatics. He employs a variety of machine learning methods to analyze large-scale biomedical datasets, using mathematical and statistical approaches to tackle pressing bioinformatics challenges.
Past Fellows

Roland Neil
Department of Criminology
Roland Neil is a sociologist who uses machine learning algorithms, geospatial analysis, and semi-parametric models to study crime and the criminal justice system. His work focuses on using large administrative datasets and advanced methods of data science to address inferential challenges faced when studying racial disparities and discrimination in policing and the life course origins of offending and criminal justice contact. His recent papers appear in the Proceedings of the National Academy of Sciences, the American Journal of Sociology, and the Annual Review of Criminology. He holds a PhD in sociology from Harvard University.
Roland is now a policy expert at the RAND Corporation.

Ivan Simpson-Kent
Department of Psychology
Ivan Simpson-Kent received his Ph.D. in Medical Science from Cambridge University in 2021. Prior to his Ph.D., Dr. Simpson-Kent was a Fulbright Fellow in Zoology at Universität Regensburg in Germany, and received a B.S. in neuroscience with secondary major in philosophy and minor in mathematics from the University of Scranton. During his postdoctoral fellowship, Dr. Simpson-Kent is investigating the associations between environmental factors, cognition, and brain development in children. To do so, he uses methods from network science and structural equation modeling to help tease apart the myriad of complex interactions among these variables and levels of organization.
Ivan is now an Assistant Professor at Leiden University.

Dimitrios Tanoglidis
Department of Physics and Astronomy
Dimitrios Tanoglidis is an astrophysicist who is excited about developing machine learning solutions for fast and efficient analysis of large astronomical datasets coming from modern galaxy surveys. In past work, he used data from the Dark Energy Survey and machine learning to discover extremely faint galaxies, adapted an object detection model for the processing of astronomical images, and developed a neural network for automated galaxy parameter inference with uncertainty quantification. He is also interested in the broader societal impact of machine learning/AI. Dimitrios holds a Ph.D. in astrophysics from the University of Chicago, and is originally from Crete, Greece.
Dimitrios is now a Senior Algorithms & Machine Learning Scientist in Walgreens Boots Alliance’s AI Lab

Colin Twomey
Department of Biology
From “fright waves” in schooling fish to the emergence of shared vocabularies for colors in human language, Colin studies the natural algorithms underlying collective behavior in living systems. His work takes a quantitative, computational approach to understanding the flow of information between group-members, as well as to the development of new methods for inferring the determinants of group-level behavioral patterns (e.g. to identify the latent “communicative needs” for colors in different languages around the world).
Colin is now Interim Executive Director of the Data Driven Discovery Initiative.
Meeting Topics
Spring 2023
1/27 – Computational approaches to understanding inter and intragenerational changes in career mobility (Xi Song)
2/3 – Species coexistence and how we can use simple microbiology experiments and image object features to detect it (Chang-Yu Chang)
2/10 – Using benchmarking to examine race disparities in NY state sentencing, Danish heathcare, and police satisfaction (Greg Ridegway)
2/17 – Identifying and classifying acoustical markers of whale songs using Google and NOAA’s Pattern Radio (special guest from University at Buffalo, Eduardo Mercado)
2/27 – Nationalization of voters and utilization of data science methods in political science (Dan Hopkins)
3/3 – Trends in data science and predictive economics (Hanming Fang)
3/17 – Tackling complexities and knowledge gaps in climate change negotiations and how data visualizations can help (Michael Weisberg)
3/24 – Events and objects in space and time, in the mind, and in language (Sarah Lee)
3/31 – A psychometric approach to quanitfying and classifying absolute and implicit pitch memory (Karen Chow)
4/7 – Global ocean circulation – climate dynamics and big data in Earth Sciences (Segey Molodotsov)
4/14 – Transformers: The machine learning model behind ChatGPT (Dimitrios Tanoglidis)
Fall 2022
9/23 – Challenges in applying deep learning to cosmological surveys (Bhuv Jain)
9/30 – Classifying stars and galaxies (Dimitrios Tanoglidis)
10/7 – Understanding the field of data science (Vijay Balasubramian)
10/14 – Methods of inquiry in the social sciences (Roland Neil)
10/21 – Issues in interpreting and establishing significance in research findings (Eleni Katifori)
10/28 – Evaluating strength of establishing causality and scientific rigor in research (Konrad Koerding)
11/4 – Computational modeling of visual processing (David Brainard); How to locate data and applications of machine learning to do so (Kieran Murphy)
11/11 – Model for predicting police officer features and rounds discharged in gun shootings (Greg Ridgeway); Friction: a short history and discussion about sparse, messy data (Sam Dillavou)
11/18 – Efficacy of bed bug infestation disclosure (Sherrie Xie), Dementia prediction using speech features (Sunghye Cho)
12/2 – Understanding the replication crisis in research, evaluating common sense (Mark Whiting); Multi-layer network analysis in relating brain structure to exposome in children (Ivan Simpson-Kent), special visitor from U of Washington (Andrew Connolly)
12/9 – Evolution of baby names and popularity of dog breeds (Josh Plotkin); Computational principles and communicative needs that shape color naming systems in natural language (Colin Twomey)
Interested in becoming a postdoctoral fellow?
If data science is a significant part of your research and you are interested in cross-disciplinary interactions, you are invited to apply to become a Fellow of our program. Please visit our application instructions page for detailed instructions.
For more questions send us an email.